This article was originally published on September 22, 2022.
Editor’s Note: This content is contributed by Yi Shan, Vice President of AI Engineering.
Xilinx netted a double win at 2021 Computer Vision and Pattern Recognition (CVPR) and IEEE International Conference on Computer Vision (ICCV) two of the top 3 worldwide computer vision academic conferences. Both CVPR and ICCV organizations double-honored Xilinx AI products team, which is a strong recognition of the team’s technical strength and innovation in global competitions.
CVPR is a premier academic conference for computer vision. The organization accepted a Xilinx AI R&D team paper, "RankDetNet: Delving into Ranking Constraints for Object Detection." At the same time, the team won third prize in the 2021 Waymo Open Dataset Challenge, part of CVPR's autonomous driving workshop. With two consecutive years of papers selected for the top academic conference of CVPR, and the outstanding performance of the Challenge Top 3, the Xilinx AI R&D team is advancing rapidly on the road of theoretical and practical "dual engine" development.
Our paper adopts a unique approach -- a ranking-based optimization algorithm for harmoniously learning to rank and localize proposals instead of classification. The engineers explored various ranking constraints, such as global, class-specific, and IoU-guided losses. Still, these methods can't solve the issue until the ranking constraints adequately explore the relationships between samples. This method is easy to implement, compatible with mainstream detection frameworks, and is computation-free for inference.
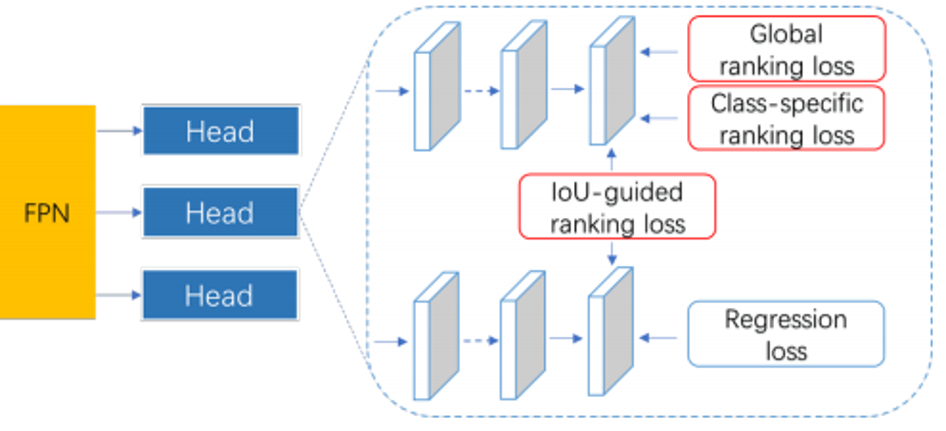
The team's algorithm replaces the conventional classification loss with three types of pair-wise ranking losses. Most important, RankDetNet achieved consistent performance improvements over 2D and 3D object detection baselines.

For the Waymo Open Dataset challenge, participants received an image and the assignment to produce a set of 3D upright boxes for the objects in the scene. The proposed model had to run faster than 70 milliseconds per frame on a Nvidia Tesla V100 GPU.
The team presented Xilinx's capability of algorithm development in advanced driver-assistance systems (ADAS) applications and chose PointPillars as a LiDAR-based backbone network to accelerate the extraction of the point cloud feature. At the same time, they used CenterPoint (anchor-free) as the detection head to improve accuracy.
The team used a structural re-parameterization technique and quality focal loss to ensure real-time performance and optimize detection performance and introduced the point-based feature based on the grid-based feature to improve the feature expression of the detection target. Using these methods, the group recorded 70.46 mAPH/L2 accuracy at a detection speed of 68.4ms per frame. That helped the team secure third place in the 3D point cloud detection challenge.
While at ICCV, another top computer vision academic conference the Xilinx AI R&D team were successfully selected two papers.
The first paper, Improve the quantization accuracy of low-bit networks through Bin regularization, proposes a novel weight regularization algorithm for improving low-precision network quantization. Instead of constraining the overall data distribution, we separably optimize all elements in each quantization bin to be as close to the target quantized value as possible. Such bin regularization
(BR) mechanism encourages the weight distribution of each quantization bin to be sharp and approximate to a Dirac delta distribution ideally. The proposed method achieves consistent improvements over the state-of-the-art quantization-aware training methods for different low-precision networks is validated on the mainstream image classification dataset ImageNet based on for different low-precision networks. Particularly, our bin regularization improves LSQ for 2-bit MobileNetV2 and
MobileNetV3-Small by 3.9% and 4.9% top-1 accuracy on ImageNet, respectively. Besides, it is easy-to-implement and compatible with the common quantization-aware training paradigm further improving the model quantization accuracy.
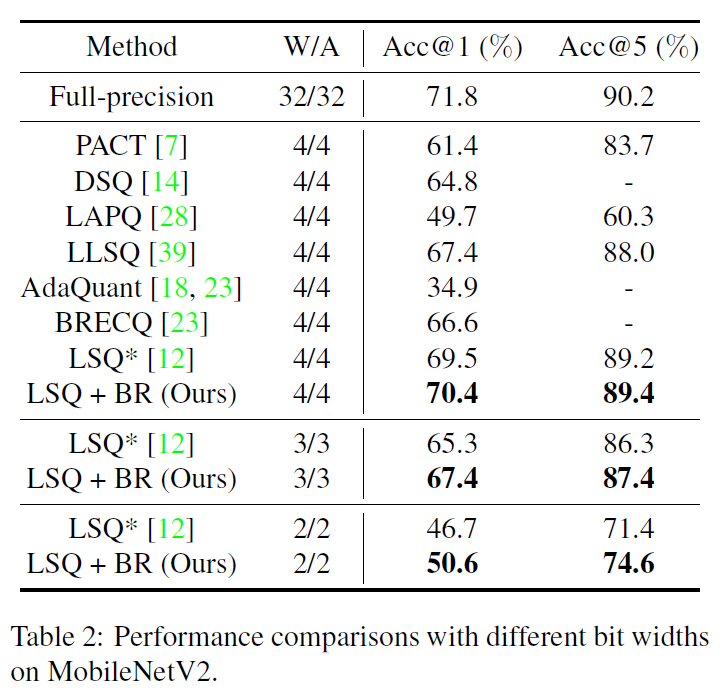
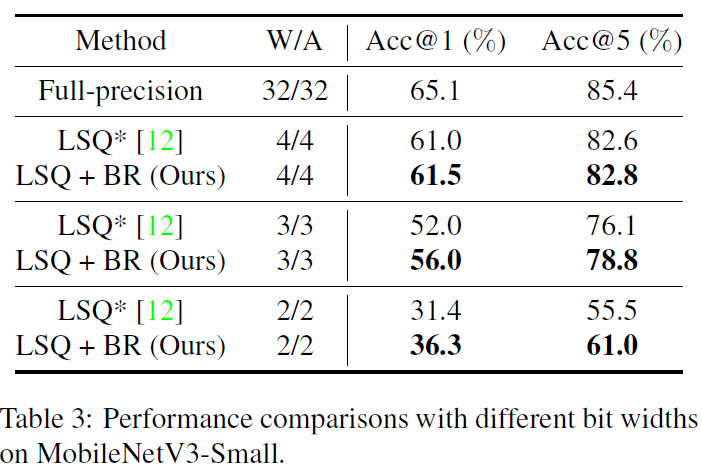
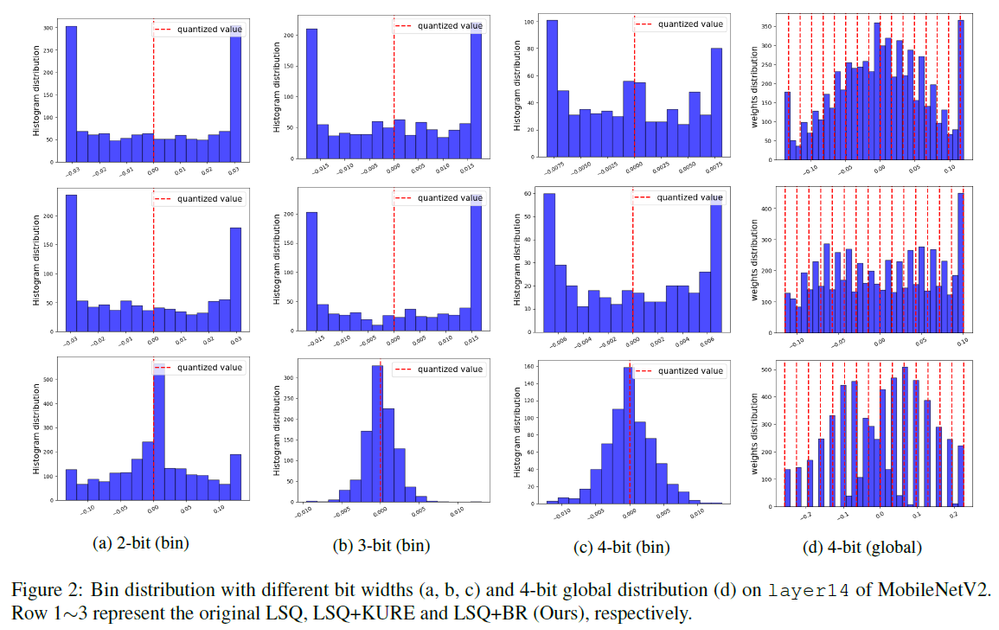
The second paper, Discriminant representation learning for unsupervised pedestrian re-identification, focuses the problems with typically two-stage training methods in pedestrian identification models. When a model that has been supervised and pre-trained in certain scenes is directly applied to a new scene, the recognition effect is greatly reduced which makes pedestrian re-identification under unsupervised domain adaption very important and can deal with the problem of no data labels in new scenes. We propose three types of technical schemes to alleviate these issues. First, we propose a cluster-wise contrastive learning algorithm (CCL) by iterative optimization of feature learning and cluster refinery to learn noise-tolerant representations in the unsupervised manner. Second, we adopt a progressive domain adaptation (PDA) strategy to gradually mitigate the domain gap between source and target data. Third, we propose Fourier augmentation (FA) for further maximizing the class separability of re-ID models by imposing extra constraints in the Fourier space. We observe that these proposed schemes are capable of facilitating the learning of discriminative feature representations. Experiments demonstrate that our method consistently achieves notable improvements over the state-of-the-art unsupervised re-ID methods on multiple benchmarks, e.g., surpassing MMT largely by 8.1%, 9.9%, 11.4% and 11.1% mAP on the Market-to-Duke, Duke-to-Market, Market-to-MSMT and Duke-to-MSMT tasks, respectively.
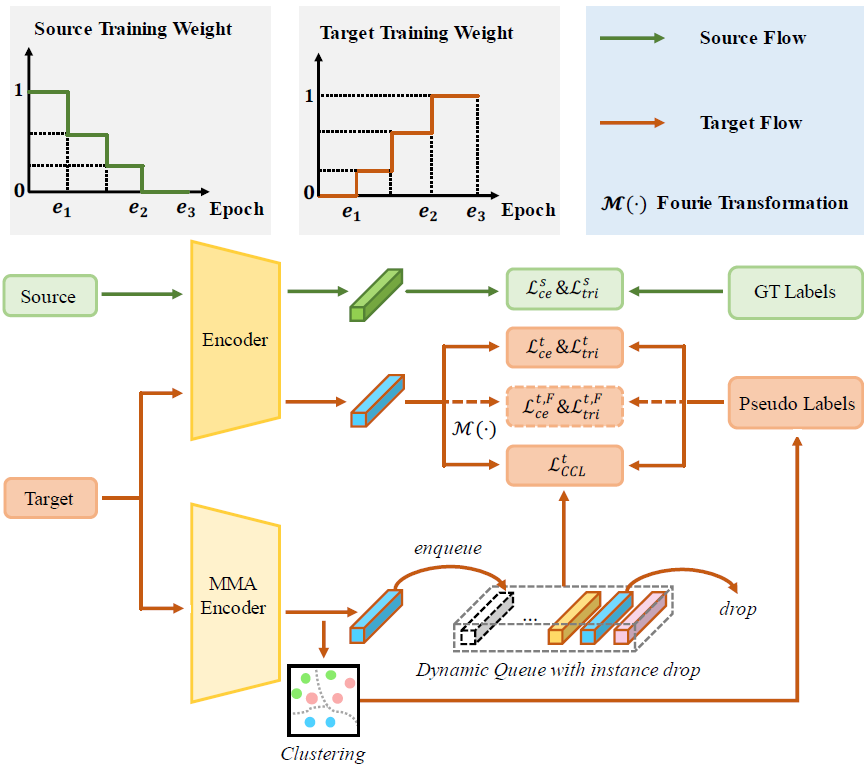
RankDetNet: Delving into Ranking Constraints for Object Detection:
Authors: Ji Liu, Dong Li, Rongzhang Zheng, Lu Tian, and Yi Shan.
Real-time 3D Detection Track Solution at the Waymo Open Dataset Challenge:
Winners: Han Liu, Rongzhang Zheng, Jinzhang Peng, and Lu Tian.
Improve the quantization accuracy of low-bit networks through Bin regularization
Authors:Tiantian Han, Dong Li, Ji Liu, Lu Tian, Yi Shan.
Discriminant representation learning for unsupervised pedestrian re-identification:
Authors: Takashi Isobe (Tsinghua University & Xilinx), Dong Li, Lu Tian, Weihua Chen (Alibaba Group), Yi Shan, Shengjin Wang (Tsinghua University)