Over the last two years, generative AI has become a major focus, with billions of people and organizations using AI tools daily. As adoption accelerates and new applications emerge, new data centers are coming online to support this transformational technology – but energy is a critical limiting factor.
In these early stages of the AI transformation, demand for compute will continue to be nearly insatiable. And in the data center, every watt of energy consumed by a chip has an impact on its energy needs, total cost of ownership, carbon emissions and, most importantly, its compute capacity. To continue driving advances in AI and broaden access, the industry must deliver higher performance and more energy efficient processors.
Driving energy efficiency through the 30x25 goal
In 2021, we announced our 30x25 goal, a vision to deliver a 30x energy efficiency improvement for AMD EPYC™ CPUs and AMD Instinct™ accelerators powering AI and high performance computing (HPC) by 2025 from a 2020 baseline. We have made steady progress against our goal by finetuning every layer from silicon to software.
Through a combination of architectural advances and software optimizations, we’ve achieved a ~28.3x[i] energy efficiency improvement in 2024 using AMD Instinct™ MI300X accelerators paired with AMD EPYC™ 9575F host CPUs, compared to the 2020 goal baseline.
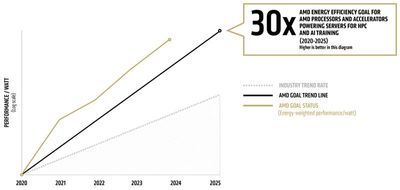
Energy efficient design starts at the architecture level
AMD takes a holistic approach to energy efficient design, balancing advancements across the many complex architectural levers that make up chip design, incorporating tight integration of compute and memory with chiplet architectures, advanced packaging, software partitions, and new interconnects. One of our primary goals across all of our products is to extract as much performance as possible while balancing energy use.
AMD Instinct MI300X accelerators pack an unprecedented 153 billion transistors and leverage advanced 3.5D CoWoS packaging to minimize communication energy and data movement overhead. With eight 5nm compute die layered on top of four 6nm IO die, all tightly connected to industry-leading 192GB of high-bandwidth memory (HBM3) capacity running at 5.2 terabytes per second, these accelerators can ingest and process massive amounts of data at an incredible pace.
Microsoft and Meta are taking advantage of these capabilities, leveraging MI300X accelerators to power key services including all live traffic on Meta’s Llama 405B models.
Memory capacity and bandwidth play a crucial role in AI performance and efficiency, and we are committed to delivering industry-leading memory with every generation of AMD Instinct accelerators. Increasing the memory on chips, improving the locality of memory access via software partitions, and optimizing how data is processed by enabling high bandwidth between chiplets can lower interconnect energy and total communication energy consumption, reducing the overall energy demand of a system. These effects multiply across clusters and data centers.
But it’s not just accelerators that impact AI performance and energy efficiency. Pairing them with the right CPU host is critical to keeping accelerators fed with data for demanding AI workloads. AMD EPYC 9575F CPUs are tailor made for GPU-powered AI solutions, with our testing showing up to 8% faster processing than a competitive CPU thanks to higher boost clock frequency[ii].
Continuous improvement with software optimizations
The AMD ROCm™ open software stack is also delivering major leaps in AI performance, allowing us to continue driving performance and energy efficiency optimizations for our accelerators well after they’ve shipped to customers.
Since we launched the AMD Instinct MI300X accelerators, we have doubled inferencing and training performance[iii] across a wide range of the most popular AI models through ROCm enhancements. We are continuously finetuning, and our engagement in the open ecosystem with partners like PyTorch and Hugging Face means that developers have access to daily updates of the latest ROCm libraries to help ensure their applications are always as optimized as possible.
With ROCm, we have also expanded support of lower abstraction AI-specific math formats including FP8, enabling greater power efficiency for AI inference and training. Leveraging lower precision math formats can alleviate memory bottlenecks and reduce latency associated with higher precision formats, allowing for larger models to be handled within the same hardware constraints, enabling more efficient training and inference processes. Our latest ROCm 6.3 release continues to extend performance, efficiency and scalability.
Where do we go from here?
Our high-performance AMD EPYC CPUs and AMD Instinct accelerators are powering AI at scale, uncovering incredible insights through the world’s fastest supercomputers, and enabling data centers to do more in a smaller footprint. We are not taking our foot off the gas – we are continuing to push the boundaries of performance and energy efficiency for AI and high performance computing through holistic chip design. What’s more, our open software approach enables us to harness the collective innovation across the open ecosystem to drive performance and efficiency enhancements on a consistent and frequent cadence.
With our thoughtful approach to hardware and software co-design, we are confident in our roadmap to exceed the 30x25 goal and excited about the possibilities ahead, where we see a path to massive energy efficiency improvements within the next couple of years.
As AI continues to proliferate and demand for compute accelerates, energy efficiency becomes increasingly important beyond the silicon, as we broaden our focus to address energy consumption at the system, rack, and cluster level. We look forward to sharing more on our progress and what’s after 30x25 when we wrap up the goal next year.
Sam Naffziger, SVP, AMD Corporate Fellow, and Product Technology Architect
[i] EPYC-030B Calculation includes 1) base case kWhr use projections in 2025 conducted with Koomey Analytics based on available research and data that includes segment specific projected 2025 deployment volumes and data center power utilization effectiveness (PUE) including GPU HPC and machine learning (ML) installations and 2) AMD CPU and GPU node power consumptions incorporating segment-specific utilization (active vs. idle) percentages and multiplied by PUE to determine actual total energy use for calculation of the performance per Watt.
28.3x is calculated using the following formula: (base case HPC node kWhr use projection in 2025 * AMD 2024 perf/Watt improvement using DGEMM and TEC +Base case ML node kWhr use projection in 2025 *AMD 2024 perf/Watt improvement using ML math and TEC) /(2020
perf/Watt * Base case projected kWhr usage in 2025). For more information, www.amd.com/en/corporate-responsibility/data-center-sustainability.
[ii] 9xx5-056A: Llama3.1-70B inference throughput results based on AMD internal testing as of 09/24/2024.
Llama3.1-70B configurations: vLLM 0.6.1.post2, TP8 Parallel, FP8, continuous batching, results in tokens/second.
2P AMD EPYC 9575F (128 Total Cores) with 8x AMD Instinct MI300X-NPS1-SPX-192GB-750W, GPU Interconnectivity XGMI, ROCm™ 6.2.0-66, 2304GB 24x96GB DDR5-6000, BIOS 1.0, Ubuntu® 22.04.4 LTS, kernel 5.15.0-72-generic
2P Intel Xeon Platinum 8592+ (128 Total Cores) with 8x AMD Instinct MI300X-NPS1-SPX-192GB-750, GPU Interconnectivity XGMI, ROCm 6.2.0-66, 2048GB 32x64GB DDR5-4400, BIOS 2.0.4, Ubuntu 22.04.4 LTS, kernel 5.15.0-72-generic
Input/Output Tokens, 2K MI300X Turin MI300X EMR Turin vs. EMR
128/1, 500 prompts 185.7 158.62 1.171
128/128, 500 prompts 6859.35 6252.68 1.097
128/2048, 500 prompts 8763.52 8048.48 1.089
2048/1, 2000 prompts 13.08 13 1.00
2048/128, 2000 prompts 1421.79 1393.54 1.02
2048/2048, 2000 prompts 6508.68 6026.48 1.08
Geomean of Relative Performance 1.076
Results may vary due to factors including system configurations, software versions and BIOS settings.
[iii] Testing conducted by internal AMD Performance Labs as of September 29, 2024 inference performance comparison between ROCm 6.2 software and ROCm 6.0 software on the systems with 8 AMD Instinct™ MI300X GPUs coupled with Llama 3.1-8B, Llama 3.1-70B, Mixtral-8x7B, Mixtral-8x22B, and Qwen 72B models.
ROCm 6.2 with vLLM 0.5.5 performance was measured against the performance with ROCm 6.0 with vLLM 0.3.3, and tests were performed across batch sizes of 1 to 256 and sequence lengths of 128 to 2048.
Configurations:
1P AMD EPYC™ 9534 CPU server with 8x AMD Instinct™ MI300X (192GB, 750W) GPUs, Supermicro AS-8125GS-TNMR2, NPS1 (1 NUMA per socket), 1.5 TiB (24 DIMMs, 4800 mts memory, 64 GiB/DIMM), 4x 3.49TB Micron 7450 storage, BIOS version: 1.8, , ROCm 6.2.0-00, vLLM 0.5.5, PyTorch 2.4.0, Ubuntu® 22.04 LTS with Linux kernel 5.15.0-119-generic.
vs.
1P AMD EPYC 9534 CPU server with 8x AMD Instinct™ MI300X (192GB, 750W) GPUs, Supermicro AS-8125GS-TNMR2, NPS1 (1 NUMA per socket), 1.5TiB 24 DIMMS, 4800 mts memory, 64 GiB/DIMM), 4x 3.49TB Micron 7450 storage, BIOS version: 1.8, ROCm 6.0.0-00, vLLM 0.3.3, PyTorch 2.1.1, Ubuntu 22.04 LTS with Linux kernel 5.15.0-119-generic.
Server manufacturers may vary configurations, yielding different results. Performance may vary based on factors including but not limited to different versions of configurations, vLLM, and drivers. MI300-62