AI algorithms are pervasive and becoming an integral part of our daily lives, from pruning junk email from inboxes or suggesting what movies one may be interested in. AI is at an inflection point in the semiconductor industry, and AMD has accelerated its focus on innovative AI solutions.
Generational Uplifts using AMD ZenDNN
Several AMD customers using complex AI inference engines are already taking advantage of the high performance offered by 4th Gen AMD EPYC processors along with targeted software optimizations via the AMD Zen Deep Neural Network (ZenDNN) version 4.0 library to enjoy a performance uplift in select applications. ZenDNN is a library that includes APIs that implement a framework for a software implementation of neural networking concepts. These APIs are enabled, tuned, and optimized for inference on AMD EPYC processors. Targeted applications including computer vision, natural language processing (NLP), and recommender systems are integrated into popular AI frameworks, such as TensorFlow, ONNX Runtime and PyTorch. These applications great performance, as shown in the multiple benchmarks results you will see below.
This section highlights four representative AI benchmark workloads: TPCx-AI, ResNet-50, BERT-Large, and DLRM. TPCx-AI represents a broad end-to-end AI workflow, and the other three workloads represent the most common AI use cases: image classification, natural language processing, and recommendation engines. These use cases showcase the performance uplift from the tight integration of the ZenDNN 4.0 library with 4th Gen AMD EPYC processors.
TPCx-AI
The TPCx Benchmark-AI (TPCx-AI) benchmark focuses on emulating the behavior of AI workloads that are relevant in today’s datacenters and cloud environments, making these world record results important anywhere AI and AMD EPYC processors and data centers are mentioned together. AMD collaborated with Dell Technologies to post five new TPCx-AI world record results with systems powered by 4th Gen AMD EPYC processors at scale factors SF3, SF10, SF30, SF100, and SF300.[1] The results at scale factors SF3, SF30, SF100, and SF300 are the industry’s first-ever results, and the SF10 results was the industry’s best result. These records represent the leading-edge performance that 4th Gen AMD EPYC processors bring to bear for the AI market. Please note that the performance shown in Figure 1 reflects several different system configurations, which are described in AI/ML Performance Highlights.
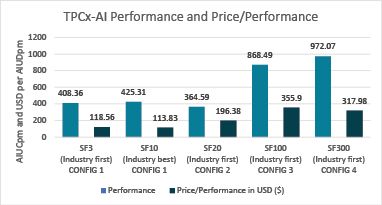
Figure 1: AMD EPYC TPCx-AI performance and price/performance
ResNet-50
ResNet is short for Residual Neural Network. It is described as a type of Artificial Neural Network (ANN) for image classification and/or a Convolutional Neural Network (CNN) specifically used for computer vision. ResNet-50 is a variant of the general ResNet that has 50 layers. It is used for image classification and training using an image dataset, such as ImageNet, before the trained model can be used for inference. AMD ran the resnet50_fp32_pretrained_model.pb (FP32) model on two systems. This model won the 2015 ImageNet competition and is commonly used to classify images. As shown below, the 4th Gen AMD EPYC system processed ~919.52 images per second with a batch size of 640 and ~927.42 images per second with a batch size of 960, a generational performance uplift of ~2.10x and ~2.09x over the 3rd Gen AMD EPYC system, respectively. The results shown in Figure 2 are the average of three runs.[2]
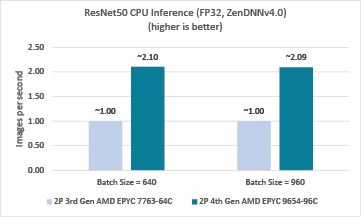
Figure 2: ResNet-50 generational performance uplift using AMD ZenDNN 4.0
BERT-Large
The Bidirectional Encoder Representations for Transformers (BERT) is a deep learning model used for various natural language processing tasks. BERT-large is a variant of BERT with 340 million parameters, pre-trained using a very large corpus of unlabeled text from the entire Wikipedia and Book Corpus and requires additional tuning for specific tasks. AMD engineers ran the wwm_uncased_L-24_H-1024_A-16 (FP32) model on the systems described above to evaluate the relative performance of the 3rd and 4th Gen AMD EPYC systems. As shown below, the 4th Gen AMD EPYC system processed ~28.74 samples per second (sequence length = 256) and ~18.65 samples per second (sequence length = 384), which translates into generational performance uplifts of ~1.83x and ~1.82x over the 3rd Gen AMD EPYC system, respectively. Each of the results shown in Figure 3 are the average of three runs.[2]
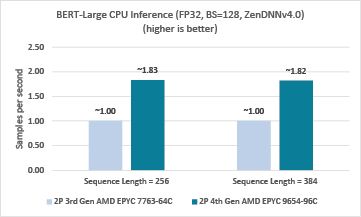
Figure 3: BERT-Large generational performance uplift using AMD ZenDNN 4.0
DLRM
Deep Learning Recommendation Model (DLRM) is an open-source model from Meta. DLRM uses a Multi-Layer Perceptron (MLP) with input, hidden, and output layers. It supports both the PyTorch and Caffe2 frameworks and is part of the popular MLPerf™ Inference benchmark suite. This recommender system from Facebook uses machine learning to make recommendations based on Facebook research. AMD engineers ran the MLPerf™ DLRM models, tb00_40M.pt (90GB FP32). As shown below, these unofficial and unpublished results show that the 4th Gen AMD EPYC system processed ~2948.38 samples per second at a batch size of 1 and ~3132,42 samples per second at a batch size of 2, a generational performance uplift of ~1.72x and ~1.83x over the 3rd Gen AMD EPYC system, respectively. The results shown in Figure 4 are the average of three runs.[2] Please note: The MLPerf™ trademark is a registered and unregistered trademark and service mark of MLCommons Association in the United States and other countries. All rights reserved. Unauthorized use strictly prohibited. Further, these results have not been verified by the MLCommons Association.
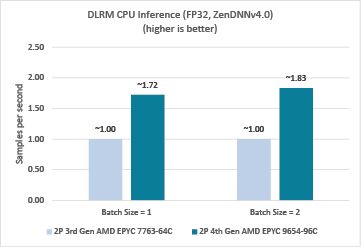
Figure 4: DLRM generational performance uplift using AMD ZenDNN 4.0
Generational Performance Uplifts
As shown in Figure 5, 4th Gen AMD EPYC processors demonstrate impressive generational performance uplifts on the following AI/ML CPU-based inference workloads:
- ResNet50: AMD engineers ran the pretrained ResNet-50v1.5 DeepSparse INT8 model from Neural Magic on multiple platforms to evaluate its CPU-only inference performance with ImageNet.[2]
- BERT Large: AMD ran the pretrained BERT-large DeepSparse INT8 model from Neural Magic on multiple platforms to evaluate its CPU-only inference performance on answering questions using the Stanford Question Answer Database (SQuAD).[2]
- Yolo v5: You Only Look Once (YOLO) is a fast, accurate object detection algorithm that divides images into a grid where each grid cell is responsible for detecting objects within itself. We ran the pretrained YOLOv5 DeepSparse INT8 model from Neural Magic on multiple platforms to evaluate its CPU-only inference performance with Common Objects in COntext (COCO).[2]
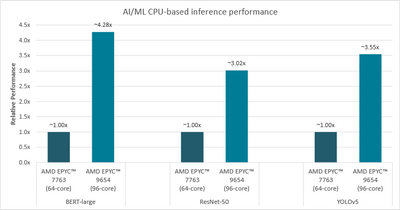
Figure 5: AI/ML Generational performance uplifts
Conclusion
The range of performance results presented in this blog showcase the AI vertical and demonstrate the breadth of coverage that 4th Gen AMD EPYC processors present to our customers for their AI workloads.
Raghu Nambiar is a Corporate Vice President of Data Center Ecosystems and Solutions for AMD. His postings are his own opinions and may not represent AMD’s positions, strategies, or opinions. Links to third party sites are provided for convenience and unless explicitly stated, AMD is not responsible for the contents of such linked sites and no endorsement is implied.
References
- The TPCx-AI results are posted at:
- Please see https://www.amd.com/system/files/documents/amd-epyc-9004-pb-aiml.pdf for detailed test information.